Executive Summary
A prominent law firm sought a centralized repository of partner information, aggregated from multiple internal and external websites. Our team delivered a fully automated solution that:
1. Extracted Data with a Python 3.9 + BeautifulSoup 4 web crawler, enriched with libraries like Requests and Selenium for dynamic content.
2. Transformed and Loaded the data into a secure, scalable AWS-based infrastructure (S3, Glue, Redshift) via an automated CI/CD pipeline.
3. Built a Retrieval-Augmented Generation (RAG) architecture leveraging LangChain, with vector embeddings stored in MongoDB’s VectorStore for advanced semantic querying.
This end-to-end system consolidated scattered partner data, unlocked complex NLP-driven queries, and significantly accelerated the firm’s research capabilities.
1. Business Context and Challenges
1.1 Fragmented Data Ecosystem
• Diverse Sources: Partner profiles resided on the firm’s site, industry directories, and legal ranking websites (e.g., Chambers, LexisNexis).
• Low Data Quality: Reliance on manual copying/pasting and ad-hoc spreadsheets increased data inconsistency and errors.
• Complex Inquiries: Existing search tools were limited to keyword matching, making it difficult to answer deeper queries like “Which partner has negotiated the largest arbitration in the energy sector?”
1.2 Strategic Objectives
• Centralized Data: Consolidate partner information into a single “source of truth.”
• Automated Pipeline: Eliminate manual overhead through robust data ingestion, transformation, and storage processes.
• Intelligent Search: Leverage modern NLP (through Large Language Models) to enable advanced retrieval and summarization.
2. Technical Approach and Methodology
2.1 Data Collection with Python 3.9, BeautifulSoup 4, and Selenium
2.1.1 Crawler Design
• Libraries and Frameworks:
• Requests for straightforward GET/POST calls.
• BeautifulSoup 4 to parse static HTML pages.
• Selenium for dynamic content extraction where JavaScript-driven elements are present (e.g., partner bios behind AJAX calls).
• Modular Architecture:
• Config Files: Each target website had a dedicated config specifying URL patterns, HTML selectors, and pagination rules.
• Error Handling: Implemented Python try-except blocks to handle broken links, timeouts, and captchas.
2.1.2 JSON Conversion
• Schema Definition: Leveraged Python’s dataclasses or pydantic to enforce a consistent schema (e.g., name, position, specialty, bio, etc.).
• Data Validation: Used built-in validators to ensure mandatory fields (e.g., email, phone number) are not null and follow standard formats.
• Output Storage: Exported validated data to JSON, then stored locally before upload to AWS S3.
2.2 ETL Pipeline with AWS and Supporting Tools
2.2.1 Data Ingestion into Amazon S3
• S3 Bucket Configuration:
• Versioning enabled for audit trails.
• Lifecycle Policies for transitions to infrequent access and archiving.
• Security: Employed AWS KMS for server-side encryption (SSE-KMS), restricting keys through IAM roles.
2.2.2 AWS Glue for Transformation
• Glue Crawlers: Automated schema discovery for JSON, generating a Glue Data Catalog.
• Glue ETL Jobs: Written in PySpark to perform:
• Cleansing: Standardize date formats, unify naming conventions (e.g., “Senior Partner” vs. “Sr. Partner”).
• Enrichment: Cross-referenced external data (e.g., Chambers ranking) to add partner accolades.
• Job Orchestration: AWS Step Functions or Apache Airflow (running on Amazon MWAA) for scheduling and dependency management.
2.2.3 Loading into Amazon Redshift
• Redshift Provisioning:
• Cluster Type: dc2.large or ra3 nodes for scalable compute and storage.
• Subnet Groups in a private VPC for secure data access.
• Data Warehouse Schema:
• Star Schema: Fact tables (e.g., “Partner_Engagements”) referencing dimensional tables (“Dim_PartnerInfo,” “Dim_Specialty”).
• Metadata Tracking: Columns for last_updated, source_url for auditing.
• Performance Optimization:
• Sort Keys and Dist Keys to minimize data movement for heavy queries.
• Column Encoding chosen by Redshift’s ANALYZE COMPRESSION function.
2.3 CI/CD and DevOps
• Version Control: GitLab for code repositories (crawler scripts, Glue ETL jobs, and Redshift schema definitions).
• Continuous Integration: GitLab CI jobs running lint checks (Flake8, Black) and unit tests.
• Docker Containerization: Packaged the crawler code into Docker images stored on AWS ECR for reproducible runs.
• Infrastructure as Code: Deployed S3 buckets, Glue jobs, and Redshift clusters via AWS CloudFormation or Terraform.
2.4 Building an NLP-Powered Search Engine (RAG) with LangChain
2.4.1 Vector Embedding Generation
• LangChain Integration:
• Language Model: Hugging Face Transformers (e.g., sentence-transformers/all-MiniLM-L6-v2) for embedding generation.
• Embedding Pipelines: Batch processed partner bios and specialized legal documents.
• Data Flow:
1. Query Redshift for partner data.
2. Use LangChain to transform text fields into vector embeddings.
3. Store embeddings in MongoDB’s VectorStore.
2.4.2 MongoDB VectorStore
• Schema:
• _id: Unique partner identifier or doc ID.
• embedding: High-dimensional float array.
• metadata: Additional fields (e.g., partner name, practice area).
• Similarity Search:
• Cosine Similarity: Implemented within the VectorStore to find nearest neighbors in embedding space.
• Indexing: Utilized MongoDB Atlas Search for indexing vectors and accelerating queries.
2.4.3 RAG Query Flow
1. User Query: Input captured via a React/Next.js web front-end or a chatbot interface built in Streamlit.
2. LangChain Orchestration:
• Retrieval: Identifies top-k relevant partner embeddings from MongoDB.
• Contextual Assembly: Aggregates relevant partner details.
• LLM Response: Passes context to the LLM (OpenAI GPT-4 or local LLM) to generate a final, succinct answer.
3. Answer Delivery: Renders a final text response along with relevant partner profiles.
3. Advanced Architecture Diagram
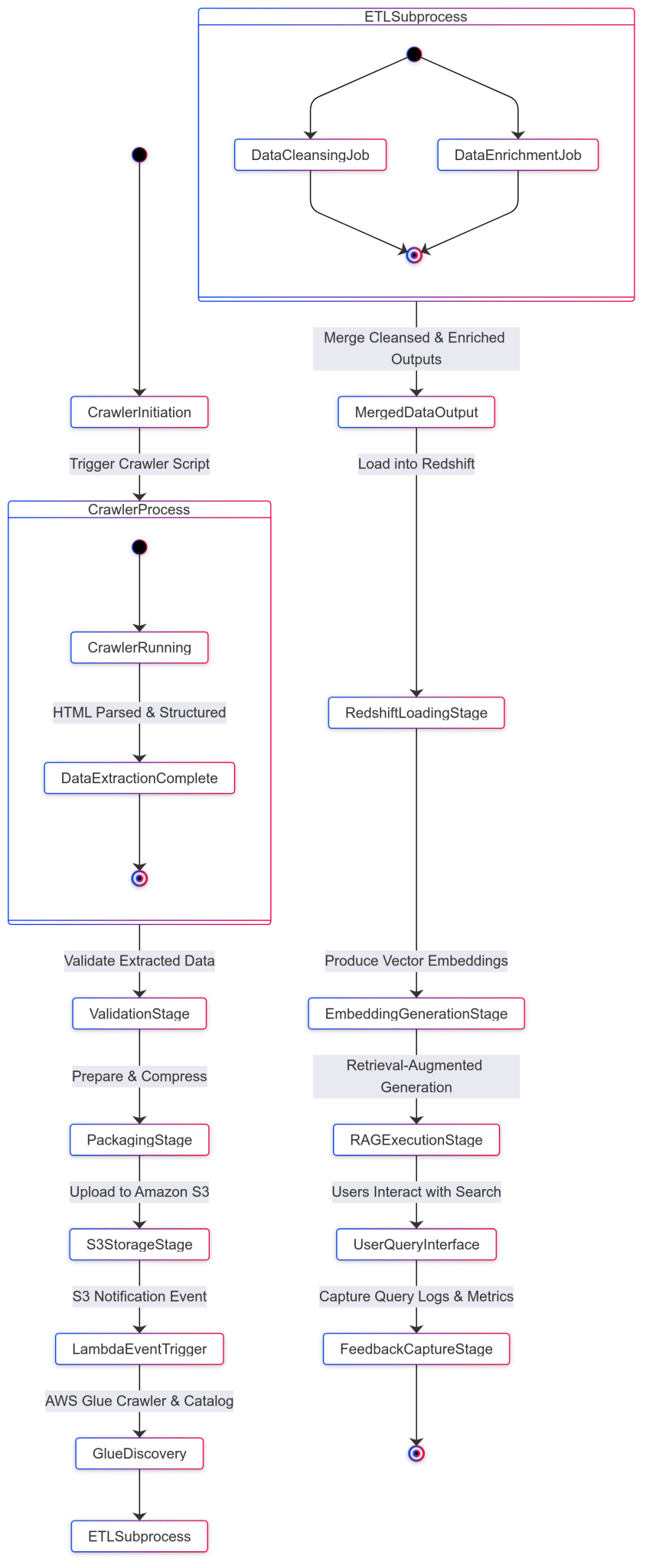
1. Crawler uses Python + Selenium to handle dynamic sites.
2. S3 houses raw JSON data, secured and versioned.
3. Glue orchestrates ETL: auto-discovery, cleaning, and loading.
4. Redshift acts as the central data warehouse for analytics.
5. LangChain + Embeddings pipeline transforms partner text data into vector forms.
6. MongoDB VectorStore enables fast semantic search.
7. LangChain RAG provides context-aware retrieval and LLM-driven answers.
8. UI/Chatbot is the front-end portal for end-users.
4. Results and Key Metrics
1. Data Quality Improvement: Null and duplicate records reduced by 90% through schema validation and automated pipeline checks.
2. Operational Efficiency: The law firm saved an estimated 800 person-hours per quarter by retiring manual data collection processes.
3. Enhanced Query Capabilities: Complex, multi-faceted queries (e.g., “List partners with arbitration experience in the APAC region”) are now answered in under 2 seconds via the RAG-based approach.
4. Scalability: The combination of AWS Glue and Redshift ensures easy horizontal scaling, accommodating thousands of new partner records and documents per day.